System dynamics does not impose models on people for the first time—models are already present in everything we do. One does not have a family or corporation or city or country in one’s head. Instead, one has observations and assumptions about those systems. Such observations and assumptions constitute mental models, which are then used as a basis for action.
The ultimate success of a system dynamics investigation depends on a clear initial identification of an important purpose and objective. Presumably a system dynamics model will organize, clarify, and unify knowledge. The model should give people a more effective understanding about an important system that has previously exhibited puzzling or controversial behavior. In general, influential system dynamics projects are those that change the way people think about a system. Mere confirmation that current beliefs and policies are correct may be satisfying but hardly necessary, unless there are differences of opinion to be resolved. Changing and unifying viewpoints means that the relevant mental models are being altered.
Unifying Knowledge
Complex systems defy intuitive solutions. Even a third-order, linear differential equation is unsolvable by inspection. Yet, important situations in management, economics, medicine, and social behavior usually lose reality if simplified to less than fifth order nonlinear dynamic systems.
Attempts to deal with nonlinear dynamic systems using ordinary processes of description and debate lead to internal inconsistencies. Underlying assumptions may have been left unclear and contradictory, and mental models are often logically incomplete. Resulting behavior is likely to be contrary to that implied by the assumptions being made about underlying system structure and governing policies.
System dynamics modeling can be effective because it builds on the reliable part of our understanding of systems while compensating for the unreliable part. The system dynamics procedure untangles several threads that cause confusion in ordinary debate: underlying assumptions (structure, policies, and parameters), and implied behavior. By considering assumptions independently from resulting behavior, there is less inclination for people to differ on assumptions (on which they actually can agree) merely because they initially disagree with the dynamic conclusions that might follow.
If we divide knowledge of systems into three categories, we can illustrate wherein lie the strengths and weaknesses of mental models and simulation models (see “Three Categories of Information”). The top of the figure represents knowledge about structure and policies; that is, about the elementary parts of a system. This is local non-dynamic knowledge. It describes information available at each decision-making point. It identifies who controls each part of a system. It reveals how pressures and crises influence decisions. In general, information about structure and policies is far more reliable, and is more often seen in the same way by different people, than is generally assumed. It is only necessary to dig out the information by using system dynamics insights about how to organize structural information to address a particular set of dynamic issues.
THREE CATEGORIES OF INFORMATION
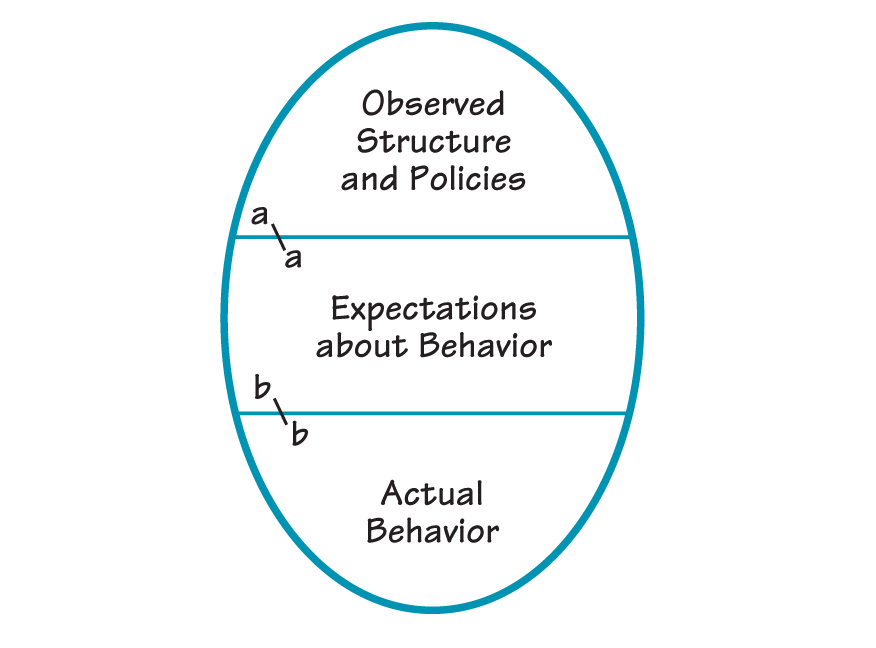
There are three categories of information about a system: knowledge about structure and policies; assumptions about how the system will behave based on the observed structure and policies; and the actual system behavior as it is observed in real life. The usual discrepancy is across the boundary a-a: expected behavior is not consistent with the known structure and policies in the system.
The middle of the figure represents assumptions about how the system will behave, based on the observed structure and policies in the top section. This middle body of beliefs are, in effect, the assumed intuitive solutions to the dynamic equations described by the structure and policies in the top section of the diagram. They represent the solutions, arrived at by introspection and debate and compromise, to the high-order nonlinear system described in the top part of the figure. In the middle lie the presumptions that lead managers to change policies or lead governments to change laws. Based on assumptions about how behavior is expected to change, policies and laws in the top section are altered in an effort to achieve assumed improved behavior in the middle section.
The bottom of the figure represents the actual system behavior as it is observed in real life. Very often, actual behavior differs substantially from expected behavior. In other words, discrepancies exist across the boundary b-b. The surprise that observed structure and policies do not lead to the expected behavior is usually explained by assuming that information about structure and policies must have been incorrect. Unjustifiably blaming inadequate knowledge about parts of the system has resulted in devoting uncounted millions of hours to data gathering, questionnaires, and interviews that have failed to significantly improve the understanding of systems.
A system dynamics investigation usually shows that the important discrepancy is not across the boundary b-b, but across the boundary a-a. When a model is built from the observed and agreed-upon structure and policies, the model usually exhibits the actual behavior of the real system. The existing knowledge about the parts of the system is shown to explain the actual behavior. The dissidence in the diagram arises because the intuitively expected behavior in the middle section is inconsistent with the known structure and policies in the top section.
UNDERINVESTMENT IN CAPACITY
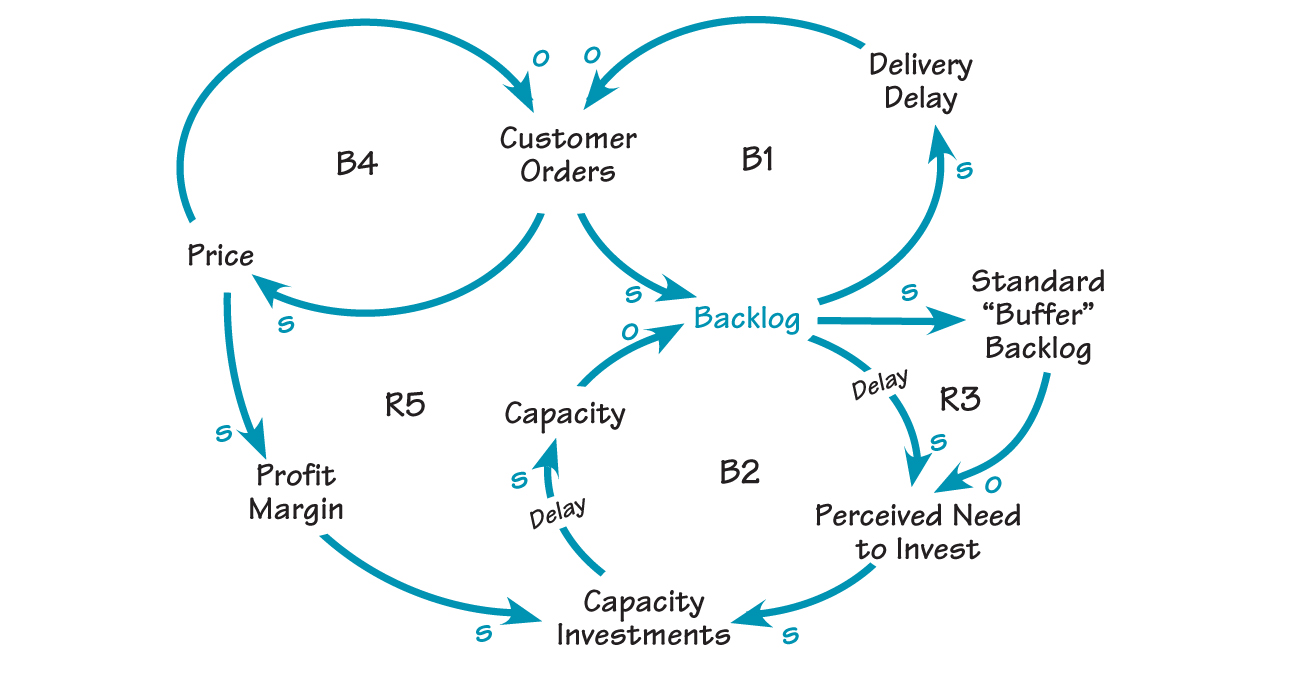
Rising backlog dampens customer orders because of increasing delivery delay (B1). However, if management is reluctant to invest in capacity expansions until the backlog reaches a certain level (Standard “Buffer” Backlog), orders will be driven down until demand equals capacity (R3). The awaited signal to expand capacity never comes, because capacity is controlling sales rather than potential demand controlling capacity (B2). If management tries lowering price to stimulate demand (B4), the resulting lower profit margins will further justify a delay in capacity investment (R5).
These discrepancies can be found repeatedly in the corporate world. A frequently recurring example in which known corporate policies cause a loss of market share and instability of employment arises from the way delivery delay affects sales and expansion of capacity (see “Underinvestment in Capacity”). Rising backlog (and the accompanying increase in delivery delay) discourages incoming orders for a product (B1) even while management favors larger backlogs as a safety buffer against business downturns. As management waits for still higher backlogs before expanding capacity, orders are driven down by unfavorable delivery delay until orders equal capacity (R3). The awaited signal for expansion of capacity never comes because capacity is controlling sales, rather than potential demand controlling capacity (B2).
When sales fail to rise because of long delivery delays, management may then lower price in an attempt to stimulate more sales (B4). Sales increase briefly but only long enough to build up sufficient additional backlog and delivery delay to compensate for the lower prices. In addition, price reductions lower profit margins until there is no longer economic justification for expansion (R5). In such a situation, adequate information about individual relationships in the system is always available for successful modeling, but managers are not aware of how the different activities of the company are influencing one another.
Lack of capacity may exist in manufacturing, product service, skilled sales people, or even in prompt answering of telephones. For example, airlines cut fares to attract passengers. But how often, because of inadequate telephone capacity, are potential customers put on “hold” until they hang up in favor of another airline?
System dynamics models have little impact unless they change the way people perceive a situation. A model must help to organize information in a more understandable way. A model should link the past to the present by showing how present conditions arose, and extend the present into persuasive alternative futures under a variety of scenarios determined by policy alternatives. In other words, a system dynamics model, if it is to be effective, must communicate with and modify the prior mental models. Only people’s beliefs—that is, their mental models—will determine action. Computer models must relate to and improve mental models if the computer models are to fill an effective role.