When encountering system dynamics modeling for the first time, sharp-minded managers often ask, “How can you have any confidence in your model if you include all those rough estimates of hard-to-measure variables?” This is perhaps one of the most important questions a decision-maker faces when considering whether and how to use system dynamics modeling. In business today, being a few percentage points off target can result in lost bonus pay, a missed promotion, or worse. So, many people naturally question a modeling process that seemingly flaunts its capability to incorporate qualitative factors at the expense of precision.
The potential for measurement error is therefore an important modeling consideration. Traditional analytical approaches often leave out qualitative factors because they are hard to measure and reduce the precision of a model’s results. But the importance of measurement error diminishes when the investigative focus shifts from concern over the system’s current state to understanding the system’s behavior over time, which is often the purpose of a system dynamics model.
Measurement Error
In traditional business analysis, conceptual (pen-and-paper) models might contain a mix of quantitative and qualitative factors, but such models rarely advance past the theoretical stage. Traditional formal (computer) models usually either bury qualitative factors within more quantitative assumptions or leave them out entirely. As a result, organizations over the years have focused much more of their analytical attention on easily measurable “hard” factors (for example, production output, lines of code, or cash flow) than on “soft” variables (for example, employee morale, efficiency of code, or customer satisfaction). But these soft factors are important components of the system structure: They can strongly influence the performance of the system. So one of the key steps to understanding dynamic social systems is crafting and using simple but explicit and sensible measures for qualitative variables.
It is important to keep in mind that any measurement will contain some degree of error. For variables that have well-established units—feet, pounds, liters, volts—the measurement error is usually a function of the accuracy and precision of the measuring device. For example, if the smallest gradation on a ruler is 1/32nd of an inch, then any measurement taken with that tool may be off by as much as 1/64th of an inch. Whether this fundamental type of error is significant depends on what is being measured and the purpose of the measurement.
For soft variables that don’t have well-established units of measure, measurement error arises from two additional sources: the definition of a unit of measure and the creation of a measurement tool. For instance, when trying to measure customer satisfaction, we might invent a unit of measure, such as the customer satisfaction index. Through survey instruments, focus groups, and other tools, we could then come up with a number to represent our best estimate of current, actual customer satisfaction. Both of these steps introduce the potential for error, in addition to the fundamental error described above. And people often cite these additional opportunities for error as justification for excluding qualitative variables from a computer modeling effort.
The Dangers of Excluding Qualitative Variables
Although qualitative factors are generally more prone to measurement error than quantitative variables, we shouldn’t exclude them on that basis alone. How well a model recreates the system’s performance—and thereby the model’s usefulness—depends on much more than measurement precision.
Another perspective is that measurement error is an important but usually static source of error in models. For that reason, the measurement error in one time period does not affect (or has a limited effect on) the measurement error in the next. For instance, the measurement error associated with this month’s plant utilization, operating hours divided by total hours in the month, is not affected by last month’s measurement error, nor will it affect next month’s. Also, well-designed measurement standards are unbiased: They tend to overestimate values as often as they underestimate them. A static and unbiased measurement error will have a relatively small impact on the depiction of a system’s dynamic behavior (behavior over time). Leaving out a qualitative factor entirely, on the other hand, means potentially omitting an influential feedback loop, and thereby creating a dynamic source of model error. Dynamic errors, unlike measurement errors, compound over time, causing the model to lose validity very quickly.
For example, consider the variable “Employee Morale” (see “Interacting Hard and Soft Variables”). As profitability drops, management intervention increases. In response to greater management controls, employee morale decreases, leading to a decline in productivity, quality, and, ultimately, profitability. Leaving this variable out of the model ignores serious reinforcing processes and quickly leads to unacceptable levels of total model error. Can we precisely measure employee morale and its impact on related variables? No. Are the processes described real? Absolutely. Although we can worry about whether a system dynamics model, with all its qualitative factors, is generating precise output, one thing is certain: A model that leaves soft variables out entirely is definitely off the mark.
Quantitative Scales for Qualitative Variables
If qualitative factors are so important, how do we incorporate them into a computer model? Although most managers can give a ballpark estimate of qualitative variables such as market focus, they often feel uncomfortable about encoding this kind of estimate in a computer model. But for many qualitative factors, a ballpark estimate by an experienced management team is the only data available and is usually a pretty good reflection of the system’s state. At the very least, this data is important because it represents the mental models of the managers involved in the model-building process.
Indexed Variables. The most straightforward way to capture qualitative variables in a model is to create an indexed variable. To do this, we typically set the value of the variable equal to “1” at some given point in time, usually the start of the simulation. We can then identify the factors that affect the indexed variable and establish mathematical or graphical relationships to cause the indexed variable to change over time.
For example, suppose we create an index for customer satisfaction that has a value of “1” at the outset of the simulation. Notice that we are not attempting to say that the current level of satisfaction is high or low; we are simply establishing a starting point. Next, we determine that the ratio of customers to telephone representatives is a key driver of customer satisfaction. Third, we ask what ratio (everything else being equal) would maintain customer satisfaction at “1” and what would happen if the ratio changed? For instance, if the “steady state” ratio were 200 customers per telephone representative, the management team could estimate the impact of letting the ratio slip to 300: satisfaction might fall by 20 percent. When we run the model, we will then know whether satisfaction goes up, down, oscillates, or remains constant, based on the behavior of the indexed variable at any given time and its value relative to “1.”
Formulating an explicit equation or graphical relationship between a qualitative variable and its drivers provides a management team with the opportunity to share mental models about the business and to try to achieve a mutual understanding. Through modeling, differing assumptions about the strength of such relationships can be tested.
Assessing Models
Interacting Hard and Soft Variables
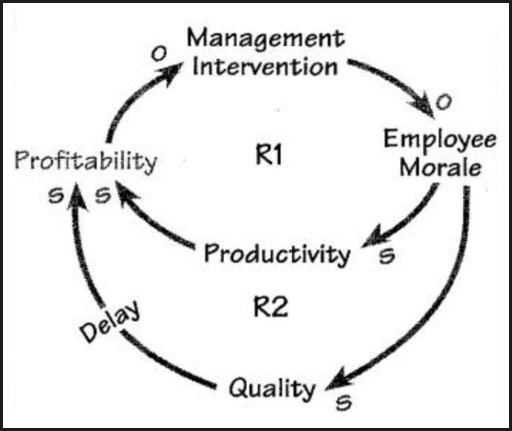
The qualitative variable “Employee Morale” Is a key component of these reinforcing loops. As profitability drops, management intervention increases. In response to greater management controls, employee morale decreases, leading to a decline in productivity, quality, and, ultimately, profitability.
If you are responsible for building and managing models, present the decision-maker with alternatives: the old way of model-building or a new way that captures the impact of qualitative variables. Use both kinds of models concurrently to explore the thinking and assumptions that went into each one, and to uncover new insights about the organization and business. See if you can move senior management from using modeling as a forecasting tool to using modeling as a way to test ideas, explore strategies, and learn how the system works.
If you are the decision-maker, ask tough questions about the assumptions going into the models you use, especially with respect to qualitative factors. For instance, ask whether employee morale and its impact on productivity are addressed in your business plan. If not, why not? Last but not least, figure out ways to free your team from the tyranny of quantitative spreadsheet thinking. Spreadsheets are an important and helpful tool, but today’s management teams need to have a variety of tools at their disposal and must know how to include qualitative factors in their thinking.
Gregory Hennessy Is co-founder and managing director of Dynamic Strategies, a collaboration of advisors working with clients to Improve organizational effectiveness, apply system dynamics, and develop organizational learning capabilities.