Avice president of a major U. S. manufacturer once questioned whether today’s rapid pace of change means that all our old tools and ways of managing are now inadequate. “Are we doomed to keep on throwing out our current tools and practices as soon as the next wave of innovations comes along?” he asked.
The answer is … “it depends.” It depends on the underlying theory on which the current tools and methods are based. If our management practices are based on transient or situation-specific phenomena, they are likely to require revision whenever the circumstances change. If, on the other hand, they are based on a structural understanding, the situation may change, but the tools will still apply.
Where Are the Cows?
Barry Richmond of High Performance Systems [now I see systems] tells this story: “While perusing a well-known economic journal, I came across an article which described a model that had been constructed to forecast U. S. milk production. The model was of the Y=f(Xi) form [Y =Y0 + a1X1 + a2X2 +…+ anXn], where the Xi’s included such things as: last year’s milk production, interest rates, spending on cattle feed, GNP growth, and other macroeconomic factors. As the article detailed, the model performed quite well as a predictive device—at least in terms of its ability to ‘track history.’ The obvious thing about this model, that would bother both dairy farmers and people who were partial to operational specifications, is: ‘where’s the cows?!’ Simply stated, if you’ve got no cows, you’ve got no milk! Crude, but true.”
How does all this talk about cows relate to our vice president’s question? Well, imagine that an epidemic swept over the country and killed all the cows. What would the above model predict for next year’s milk production? The answer would most likely look a lot like the number for last year’s milk production, which is clearly incorrect. The model must be abandoned.
“Unfair,” you might say. “It’s not that the model is wrong. It’s just that the world has changed dramatically since the model was originally built and the changes must now be added.” But what has really changed? Yes, the cows are now dead, but the basic fact that milk comes from cows, and that without cows there can be no milk, is as true now as it was before the mass decimation. From a structural perspective, the nature of the world has not changed at all. The model was inadequate because it was based on situation-specific data that has now changed.
Structural Thinking
When we look at the world through a structural lens, we are interested in understanding how things actually work. We are less interested in correlational relationships and more interested in the causal structures that produce the observed behavior. This is not to say that nonstructural models aren’t valuable. Regression models, for example, have many applications and are useful for identifying correlation, explaining sources of variance, and extrapolating from historical data. Those models are inadequate, however, for gaining insight into how a system actually operates.
MILK PRODUCTION MODEL
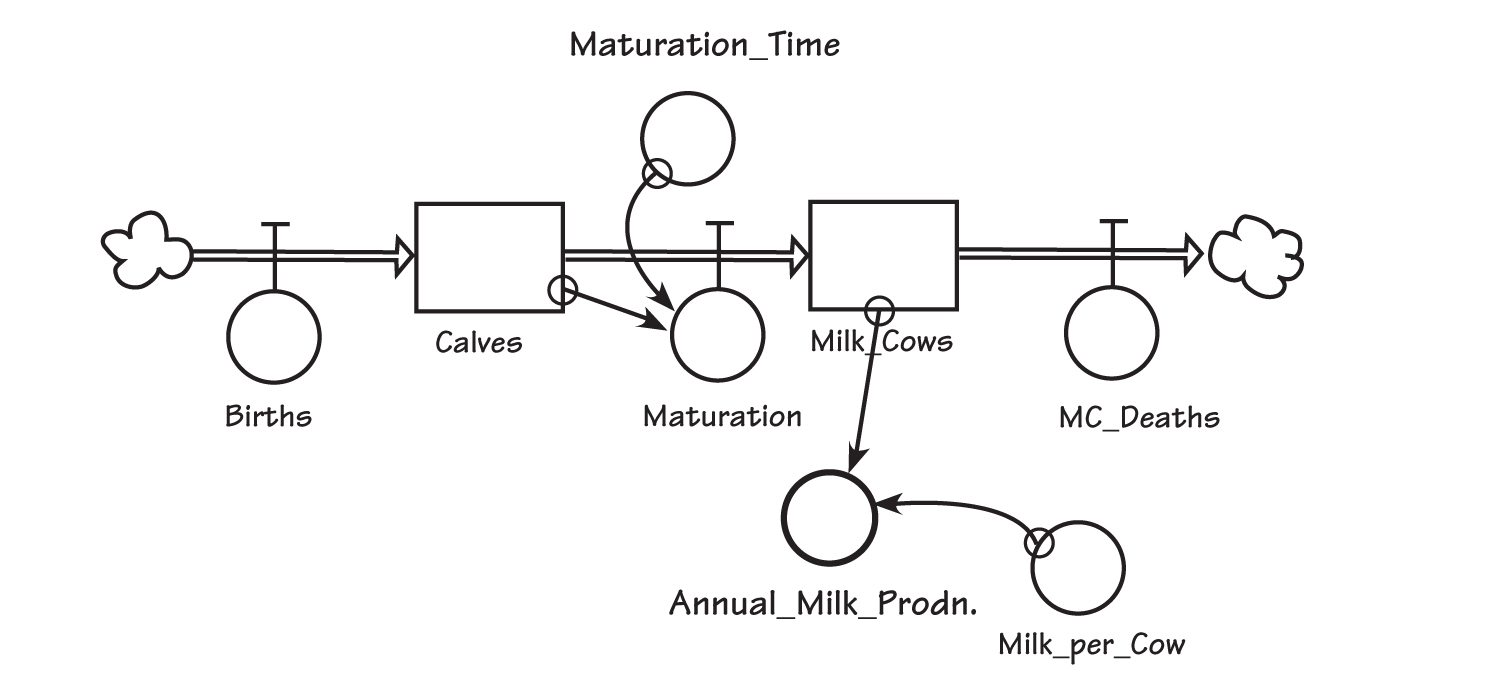
If we wanted to create a structural representation of milk production, we would begin with the central accumulator “Milk Cows.” Milk production is determined by the number of cows and the amount of milk per cow. To create our hypothetical scenario of an epidemic, we would simply enter zero for the number of milk cows. The resulting annual milk production would also be zero.
If we were to look at the milk production model from a structural viewpoint, we would start with the basic fact that milk comes from cows. Therefore, cows are the central accumulator in the model—the number of cows accumulates over time, as cows are born, mature, and become milk cows (see “Milk Production Model”).
Depending on the scope of our study, we may be interested in representing the lifecycle of all cows, or just milk cows. In this case, we will focus our attention on the flow of cows from birth through maturity into the milk cow accumulator. The annual milk production is then determined by the number of milk cows at any one time and the amount of milk per cow. Of course, there are many other factors that affect milk production, such as food supplies, milk demand, and dairy farmers. These factors could also be added to our diagram in the form of additional accumulators and flows.
The resulting model can then be simulated on a computer to see how annual milk production behaves over time. To create our hypothetical epidemic scenario, for example, we would simply put zero for the stock of cows. In that event, the annual milk production would also equal zero. Because this model is tied to the structure of the system, not just historical data, it would not have to be thrown out even if all of the cows suddenly died.
Levels of Explanation
We live in the world of events. As a result, we encounter and navigate through the rapids of life on an event by-event basis. But this does not mean that we must act on each event as if it were an isolated occurrence. We can look at patterns of behavior over time and try to glean lessons from the past that will improve our ability to handle present situations. That is the purpose of forecasting models.
Forecasting models, like the economist’s milk production model described above, attempt to provide information about the future by looking at the past. But in many ways, managing on the basis of forecasts is a lot like trying to drive a car by looking through the rearview mirror. When does it work best? When the road is straight and there are no obstacles in the way. When does it fail? The rest of the time! When using a forecasting model, you only realize you have missed a turn once you see the cliff’s edge behind you and feel the sensation of free fall hit your stomach.
Forecasting provides very little insight into what actually produces the observed behavior. Consequently, it allows us to anticipate and react to changes only if they do not deviate too much from past behavior. Models, on the other hand, capture the structural forces at work and are therefore less situation-dependent. To come back to the vice president’s question, structural thinking provides a more stable basis of understanding that will last even through times of turbulent change.
Generic Thinking Skills
SALES GROWTH MODEL
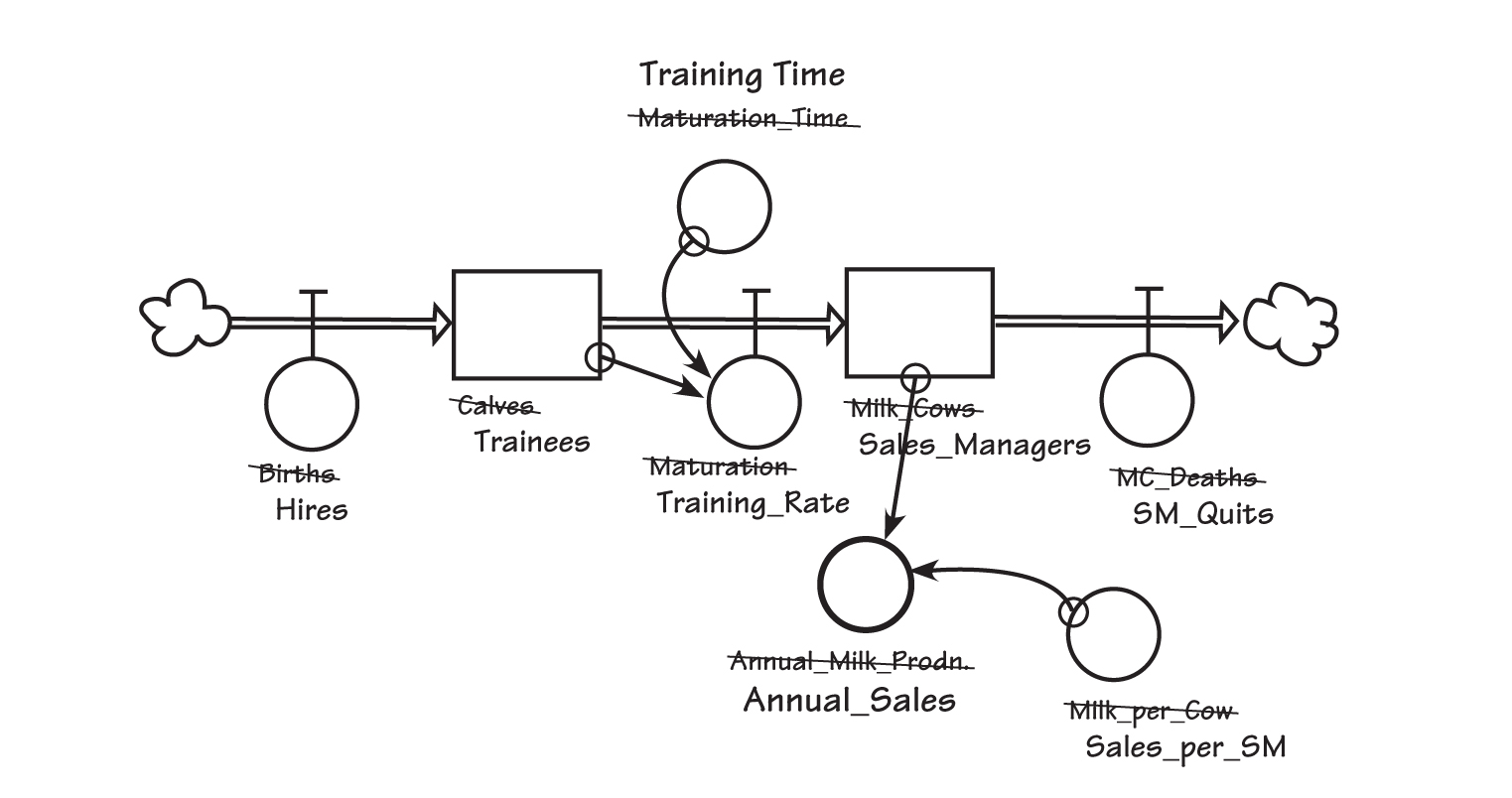
If we replace the names of the variables in the “Milk Production Model” with those listed above, we can create a model that explores sales growth. The same generic resource development structure can be used to describe both processes.
If we begin to view the world through a structural perspective, another benefit emerges—the ability to transfer insight. This ability to see similar structures occurring in diverse settings is referred to as “generic thinking,” and the structures themselves are referred to as “generic structures.”
For example, if we take the “Milk Production Model” and substitute “hires” for “births,”, “trainees” for “calves,” and “sales managers” for “milk cows,” we can transform the milk cow model into a model that can be used to explore the structural forces that influence annual sales (see “Sales Growth Model”). The same generic resource development structure underlies both models. Although we may debate whether it takes longer to produce a milk cow or a sales manager, we can agree that the structure of both processes is fundamentally the same.
Daniel H. Kim is co-founder of Pegasus Communications, founding publisher of The Systems Thinker newsletter, and a consultant, facilitator, teacher, and public speaker committed to helping problem-solving organizations transform into learning organizations.
For further reading about structural thinking and the other critical thinking skills included under the systems thinking umbrella, see Barry Richmond’s The Thinking in Systems Thinking: Seven Essential Skills (Pegasus Communications, 2000).